The Role of AI in Predicting Disease Outbreaks and Preventing Epidemics
November 11, 2024
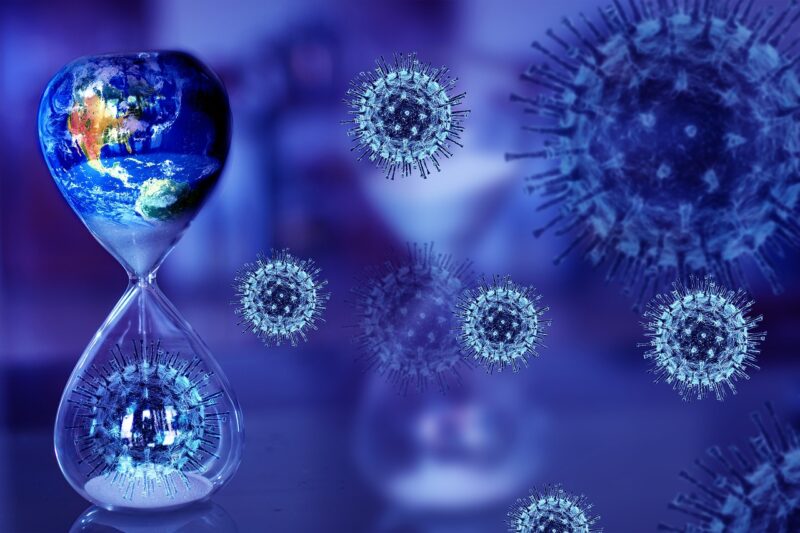
In recent years, artificial intelligence (AI) has revolutionized numerous sectors, from finance to entertainment. One of the most significant applications of AI lies in the field of public health, specifically in predicting disease outbreaks and preventing epidemics. The capability of AI to analyze vast datasets, recognize patterns, and forecast outcomes has transformed how health professionals approach disease monitoring and control.
1. Understanding the Basics of Disease Outbreaks
Disease outbreaks occur when an infectious disease spreads rapidly among a population. Understanding the factors that contribute to outbreaks requires a multifaceted approach, considering epidemiological data, environmental conditions, and human behaviors. Traditionally, health organizations relied on historical data and expert analysis to monitor and predict outbreaks. However, these methods can be limited by the speed of data collection and the inability to process complex information in real time.
In contrast, AI technologies can enhance how we understand and respond to disease outbreaks. By harnessing data from numerous sources—including hospital records, social media, climate data, and population demographics—AI algorithms can spot trends and generate real-time predictions that were previously impossible to achieve.
2. How AI Predicts Disease Outbreaks
AI’s predictive capabilities are rooted in machine learning and data analytics. Here are some key methods by which AI is used to predict disease outbreaks:
- Data Collection and Integration: AI systems can aggregate data from various sources, such as electronic health records (EHRs), geographic information systems (GIS), and climate data. The integration of this data allows for a comprehensive view of potential outbreak scenarios.
- Pattern Recognition: Machine learning algorithms are trained to identify patterns from historical outbreak data. By analyzing past outbreak cases, AI systems learn to recognize signs that may indicate an imminent outbreak.
- Forecasting Models: AI can build predictive models based on the data it processes. These models can forecast future outbreak locations, timing, and potential severity. Using techniques such as time-series analysis, AI can enhance the accuracy of predictions, allowing health officials to take preemptive measures.
The combination of these techniques allows AI to generate insights that significantly improve the prediction of disease outbreaks.
3. Real-World Applications of AI in Predicting Outbreaks
AI’s potential for predicting disease outbreaks has already been realized in various scenarios:
- Flu Surveillance: Organizations like Google Flu Trends have utilized search engine data to track flu outbreaks in real time. Through analyzing search queries, AI can estimate the spread and severity of flu season, leading to better resource allocation in healthcare settings.
- Epidemic Prediction Models: During the Ebola outbreak, AI algorithms were employed to predict the spread of the disease by analyzing travel patterns, healthcare infrastructure, and environmental factors. Organizations like IBM have also developed AI tools to enhance public health response capabilities.
- COVID-19 Monitoring: The COVID-19 pandemic highlighted AI’s role in tracking emerging diseases. Models leveraging AI have been used to predict hotspots for disease spread, analyze contact tracing data, and optimize deployment of vaccination resources. AI tools like BlueDot and the COVID-19 Mobility Data Network provided vital information to public health officials and policymakers for timely decision-making.
The effectiveness of AI in these situations demonstrates its crucial role in managing and mitigating public health crises.
4. Challenges and Limitations of AI in Disease Prediction
Despite its advantages, the implementation of AI in predicting disease outbreaks is not without challenges:
- Data Quality and Availability: The performance of AI models depends heavily on high-quality, comprehensive datasets. In many regions, especially low-income countries, data may be sparse or inconsistent, limiting the effectiveness of AI predictions.
- Algorithm Bias: AI algorithms can inadvertently learn biases present in historical data. This can lead to inaccurate predictions, especially for marginalized populations or regions lacking adequate health resources.
- Interpretability: Many AI models operate as ‘black boxes’, meaning their predictions can be challenging to understand or explain. This lack of transparency can hinder trust among healthcare professionals and policymakers who rely on these insights to make critical decisions.
Addressing these challenges requires ongoing research, collaboration across disciplines, and investment in data infrastructure and AI literacy.
5. The Future of AI in Public Health
The role of AI in predicting disease outbreaks and preventing epidemics is expected to expand in the coming years. Some areas of potential growth include:
- Real-Time Surveillance Systems: As AI technology evolves, we can anticipate more sophisticated and real-time surveillance systems capable of detecting outbreaks sooner than ever before.
- Personalized Health Insights: AI is paving the way for personalized medicine. By analyzing individual health data, AI can provide tailored recommendations for preventive measures and early detection of diseases.
- Global Collaboration: AI’s predictive capabilities will enhance worldwide collaboration in tackling public health crises. Ensuring data sharing and developing collective response strategies will be crucial in mitigating future outbreaks.
The future promises a more interconnected and responsive public health system, powered by AI advancements. Through collaboration between technologists, healthcare professionals, and policymakers, we can harness the full potential of AI to save lives and protect communities.
Conclusion
In conclusion, AI is reshaping the landscape of public health by providing unprecedented tools for predicting disease outbreaks and preventing epidemics. Its ability to analyze complex datasets, recognize patterns, and generate real-time forecasts is enhancing our capacity to respond to health crises effectively. However, stakeholders must address challenges such as data quality, algorithm bias, and transparency to fully leverage AI’s potential. As we look to the future, the integration of AI into public health practice could very well be a game-changer, leading to healthier populations and more resilient societies worldwide.