Exploring Explainable AI: How We Can Understand and Trust Machine Decisions
November 11, 2024
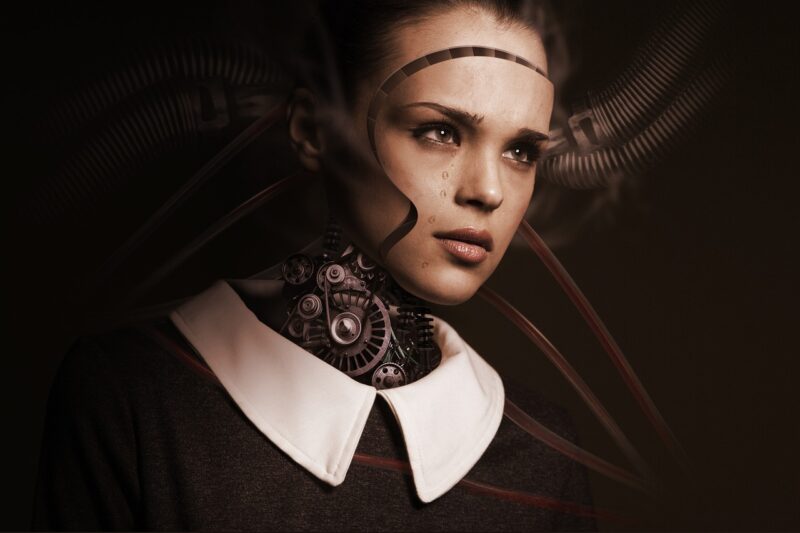
Artificial Intelligence (AI) has permeated almost every aspect of modern technology, revolutionizing industries by providing intelligent solutions that enhance efficiency and productivity. However, the very complexity of AI systems poses challenges concerning their decision-making processes and the transparency behind them. As machine learning models become increasingly sophisticated, the need for Explainable AI (XAI) has emerged as a critical area of focus. This article delves into the importance of explainability in AI, its implications for trust, and how we can bridge the gap between machine decisions and human understanding.
1. What is Explainable AI?
Explainable AI refers to methods and techniques within artificial intelligence that make the outputs and decisions of complex models understandable by humans. It aims to provide clarity on how AI systems arrive at conclusions, ensuring they operate transparently and ethically.
XAI encompasses several methodologies, including:
- Interpretable Models: Using models that are inherently understandable, such as linear regression or decision trees, rather than complex black-box systems like deep neural networks.
- Post-hoc Explanation Techniques: Techniques applied after model training that help explain model predictions, including LIME (Local Interpretable Model-Agnostic Explanations) and SHAP (SHapley Additive exPlanations).
- Visualizations: Using graphical representations to illustrate how models make decisions, highlighting key features influencing outcomes.
The growing importance of Explainable AI stems from the necessity for accountability and trust in AI systems in various domains such as finance, healthcare, and criminal justice.
2. Why is Explainability Important?
As we embrace AI in critical decision-making processes, understanding how these systems operate is vital for several reasons:
- Trust: Users are more likely to trust AI systems when they can understand how decisions are made. Explainable AI fosters trust as stakeholders can verify that systems are functioning as intended, reducing hesitance to adopt new technologies.
- Ethics and Fairness: AI models can inadvertently propagate biases if not designed and monitored correctly. XAI allows for scrutiny into decision-making processes, identifying and correcting biased outcomes, thus promoting fairness and ethics in AI applications.
- Regulatory Compliance: Governments and institutions are increasingly prioritizing the ethical use of AI. Regulations, such as GDPR in Europe, mandate that individuals have the right to explanations regarding automated decisions. XAI becomes essential for compliance with such laws.
- Improving Models: Understanding AI decision-making provides insight into model performance. Identifying areas for improvement or common pitfalls aids in developing more robust and accurate AI systems.
In summary, implementing XAI is critical for building confidence in AI systems and ensuring responsible usage across various sectors.
3. How Does Explainable AI Work?
Understanding the mechanics of XAI involves various techniques that can elucidate the decision-making process of different AI models. Here are a few methodologies employed:
- Feature Importance: This method assesses which input features significantly affect the model’s predictions. By determining which features carry the most weight in decisions, stakeholders gain clarity on how these factors interact within the model.
- Surrogate Modeling: A simpler model (like a decision tree) is trained to predict the output of a more complex black-box model. This approximation helps in interpreting complex models by providing a more understandable representation of how decisions are derived.
- Instance-based Explanations: Techniques like LIME provide localized explanations for individual predictions, clarifying how features influenced that particular decision as opposed to the global model behavior.
- Counterfactual Explanations: By showing how a slight alteration in input could lead to a different outcome, stakeholders can better grasp the decision boundary and the model’s logic.
These methodologies not only enhance transparency but also create avenues for users and stakeholders to interact meaningfully with AI systems, fostering better understanding and facilitating dialogue regarding AI’s implications.
4. The Challenges of Implementing Explainable AI
While the concept of XAI holds promise, its implementation presents several challenges:
- Trade-off Between Accuracy and Explainability: More complex models, like deep learning networks, often achieve higher accuracy but are less interpretable. Balancing the trade-off between building accurate models and ensuring their outputs are explainable is a fundamental challenge in AI development.
- Technical Complexity: The integration of XAI techniques involves technical sophistication, requiring a deep understanding of both AI algorithms and appropriate interpretation methods. Many AI practitioners may lack this necessary expertise.
- User Understanding: Stakeholders may struggle to comprehend explanations provided by complex models, necessitating further simplification which can dilute the value of explanations.
- Evolving Standards and Regulations: As AI develops, so do the expectations for explainability. Companies must stay current with evolving standards, adding pressure on organizations to continuously adapt their XAI practices.
Addressing these challenges is imperative to harness the full potential of Explainable AI while ensuring ethical and accurate decision-making in AI applications.
5. The Future of Explainable AI
As AI continues to evolve, the importance of Explainable AI will only grow. Here are a few predictions for its future:
- Integration of XAI in Education: Institutions will increasingly emphasize XAI in AI curricula, ensuring that future professionals are equipped with the skills necessary to create and interpret explainable systems.
- Automated XAI Solutions: Tools and platforms that automatically generate explanations for AI models will emerge, simplifying the process for organizations that rely on AI without the necessary in-depth expertise.
- Greater Regulatory Requirements: Governments will likely implement more stringent regulations regarding AI explainability, especially in sensitive sectors such as healthcare, finance, and law enforcement.
- Public Engagement and Discussion: The dialogue surrounding AI’s societal implications, biases, and fairness will receive more attention, fostering greater public understanding of AI technologies and their decision-making processes.
The growing focus on XAI will ensure that decision-making processes remain transparent and accountable, ultimately creating an ecosystem where trust and technology coexist harmoniously.
Conclusion
Exploring Explainable AI is not just an academic exercise but a practical necessity in our increasingly AI-driven world. As we advance in technology, clarifying how machine decisions are made fosters trust, accountability, and ethicality. By prioritizing explainability, we move towards an inclusive future where AI systems work for humanity rather than simply alongside it. The development of XAI techniques marks a significant step towards democratizing AI and ensuring its benefits are accessible, understandable, and optimized for everyone.
As we navigate this complex terrain, it is crucial for stakeholders, developers, and users to embrace the principles of Explainable AI, integrating them into the AI lifecycle and promoting systems that are both powerful and interpretable. The journey to trust in AI is ongoing, and with commitment to explainability, we’re fostering a future where technology aligns with human values and understanding.